Lead Data Scientist Interview Questions And Answers
Download Lead Data Scientist Interview Questions and Answers PDF
Strengthen your Lead Data Scientist interview skills with our collection of 60 important questions. Each question is crafted to challenge your understanding and proficiency in Lead Data Scientist. Suitable for all skill levels, these questions are essential for effective preparation. Download the free PDF now to get all 60 questions and ensure you're well-prepared for your Lead Data Scientist interview. This resource is perfect for in-depth preparation and boosting your confidence.
60 Lead Data Scientist Questions and Answers:
Lead Data Scientist Job Interview Questions Table of Contents:
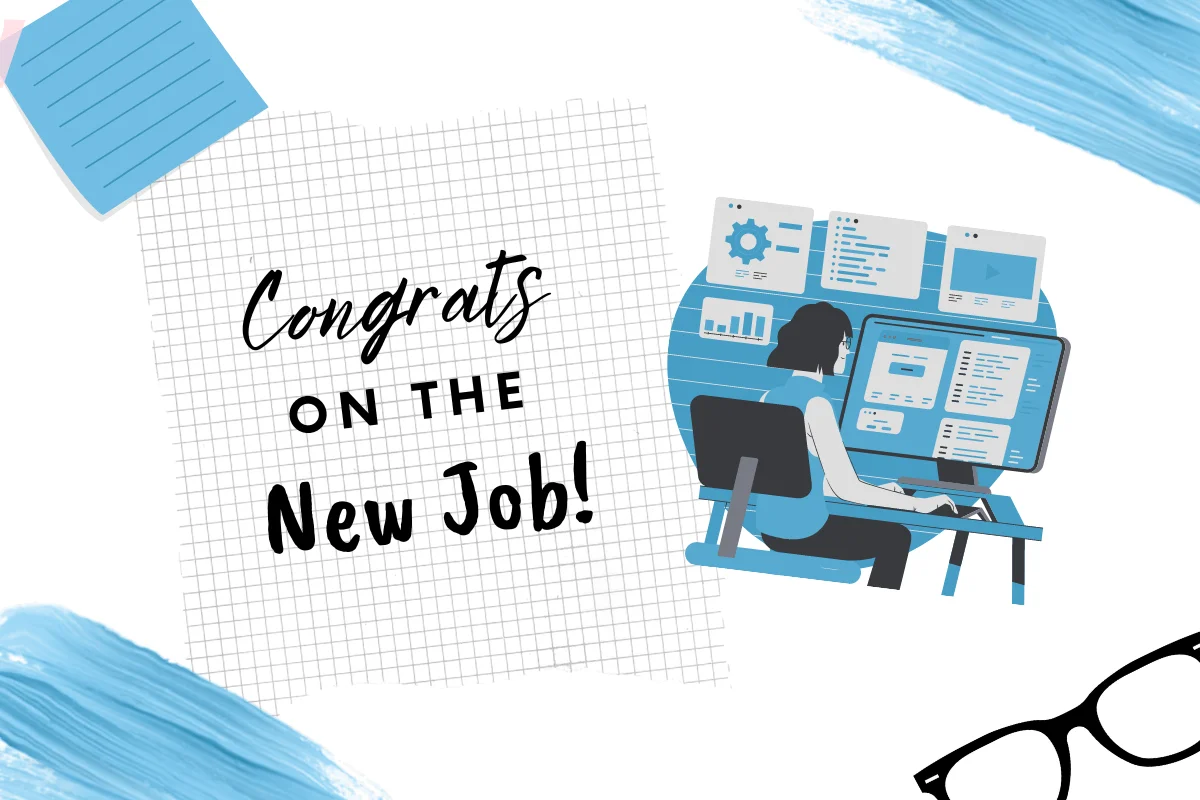
1 :: Can you write the syntax to set the path for a current working directory in R environment?
Setwd(“dir_path”)
Different syntax can be asked in R Data science interview questions.
Read MoreDifferent syntax can be asked in R Data science interview questions.
2 :: Do you know how to Merge the files into a single dataframe?
At last, we have to iterate the list of files in the current working directory. Also, we need to put them together to form a data frame. Moreover, when the script encounters the first file in the file_list, then it creates the main data frame to merge everything into. This is done using the !exists conditional:
If their dataset exists, then a temp_dataset called temporary data frame will be created and added to the dataset. Moreover, we have to delete temporary data frame. That is been removed when we’re done with it using the rm(temp_dataset) command.
If dataset doesn’t exist (!exists is true), then we have to create it.
Read MoreIf their dataset exists, then a temp_dataset called temporary data frame will be created and added to the dataset. Moreover, we have to delete temporary data frame. That is been removed when we’re done with it using the rm(temp_dataset) command.
If dataset doesn’t exist (!exists is true), then we have to create it.
3 :: Tell me how do you define big data?
It's likely that you'll be interviewed by an HR rep, an end business user, and an IT pro. Each person will probably ask you to explain what big data is, and how the data analysis discipline works with big data to produce insights.
You can start your answer with something fundamental, such as "big data analysis involves the collection and organization of data, and the ability to discover correlations between the data that provide revelations or insights that are actionable." You must be able to explain this in terms that resonate with each interviewer; the best way to do this is to illustrate the definition with an example.
Read MoreYou can start your answer with something fundamental, such as "big data analysis involves the collection and organization of data, and the ability to discover correlations between the data that provide revelations or insights that are actionable." You must be able to explain this in terms that resonate with each interviewer; the best way to do this is to illustrate the definition with an example.
4 :: Tell me what is bias, variance trade off?
Bias:
“Bias is error introduced in your model due to over simplification of machine learning algorithm.” It can lead to underfitting. When you train your model at that time model makes simplified assumptions to make the target function easier to understand.
Low bias machine learning algorithms - Decision Trees, k-NN and SVM
Hight bias machine learning algorithms - Liear Regression, Logistic Regression
Variance:
“Variance is error introduced in your model due to complex machine learning algorithm, your model learns noise also from the training dataset and performs bad on test dataset.” It can lead high sensitivity and overfitting.
Read More“Bias is error introduced in your model due to over simplification of machine learning algorithm.” It can lead to underfitting. When you train your model at that time model makes simplified assumptions to make the target function easier to understand.
Low bias machine learning algorithms - Decision Trees, k-NN and SVM
Hight bias machine learning algorithms - Liear Regression, Logistic Regression
Variance:
“Variance is error introduced in your model due to complex machine learning algorithm, your model learns noise also from the training dataset and performs bad on test dataset.” It can lead high sensitivity and overfitting.
5 :: Tell me what is Random Forest? How does it work?
Random forest is a versatile machine learning method capable of performing both regression and classification tasks. It is also used for dimentionality reduction, treats missing values, outlier values. It is a type of ensemble learning method, where a group of weak models combine to form a powerful model.
In Random Forest, we grow multiple trees as opposed to a single tree. To classify a new object based on attributes, each tree gives a classification. The forest chooses the classification having the most votes(Over all the trees in the forest) and in case of regression, it takes the average of outputs by different trees.
Read MoreIn Random Forest, we grow multiple trees as opposed to a single tree. To classify a new object based on attributes, each tree gives a classification. The forest chooses the classification having the most votes(Over all the trees in the forest) and in case of regression, it takes the average of outputs by different trees.
6 :: Do you know what regularization is and why it is useful?
Regularization is the process of adding tunning parameter to a model to induce smoothness in order to prevent overfitting. This is most often done by adding a constant multiple to an existing weight vector. This constant is often the L1(Lasso) or L2(ridge). The model predictions should then minimize the loss function calculated on the regularized training set.
Read More7 :: Explain me what are feature vectors?
A feature vector is an n-dimensional vector of numerical features that represent some object. In machine learning, feature vectors are used to represent numeric or symbolic characteristics, called features, of an object in a mathematical, easily analyzable way.
Read More8 :: Please explain me cross-validation?
It is a model validation technique for evaluating how the outcomes of a statistical analysis will generalize to an independent data set. It is mainly used in backgrounds where the objective is forecast and one wants to estimate how accurately a model will accomplish in practice. The goal of cross-validation is to term a data set to test the model in the training phase (i.e. validation data set) in order to limit problems like overfitting and gain insight on how the model will generalize to an independent data set.
Read More9 :: Tell us what are confounding variables?
These are extraneous variables in a statistical model that correlate directly or inversely with both the dependent and the independent variable. The estimate fails to account for the confounding factor.
Read More10 :: Can you please explain selective bias?
Selection bias, in general, is a problematic situation in which error is introduced due to a non-random population sample.
Read More11 :: Tell us what tools or devices help you succeed in your role as a data scientist?
This question's purpose is to learn the programming languages and applications the candidate knows and has experience using. The answer will show the candidate's need for additional training of basic programming languages and platforms or any transferable skills. This is vital to understand as it can cost more time and money to train if the candidate is not knowledgeable in all of the languages and applications required for the position. Answers to look for include:
☛ Experience in SAS and R programming
☛ Understanding of Python, PHP or Java programming languages
☛ Experience using data visualization tools
Read More☛ Experience in SAS and R programming
☛ Understanding of Python, PHP or Java programming languages
☛ Experience using data visualization tools
12 :: Tell us how would you validate a model you created to generate a predictive model of a quantitative outcome variable using multiple regression?
Proposed methods for model validation:
☛ If the values predicted by the model are far outside of the response variable range, this would immediately indicate poor estimation or model inaccuracy.
☛ If the values seem to be reasonable, examine the parameters; any of the following would indicate poor estimation or multi-collinearity: opposite signs of expectations, unusually large or small values, or observed inconsistency when the model is fed new data.
☛ Use the model for prediction by feeding it new data, and use the coefficient of determination (R squared) as a model validity measure.
☛ Use data splitting to form a separate dataset for estimating model parameters, and another for validating predictions.
☛ Use jackknife resampling if the dataset contains a small number of instances, and measure validity with R squared and mean squared error (MSE).
Read More☛ If the values predicted by the model are far outside of the response variable range, this would immediately indicate poor estimation or model inaccuracy.
☛ If the values seem to be reasonable, examine the parameters; any of the following would indicate poor estimation or multi-collinearity: opposite signs of expectations, unusually large or small values, or observed inconsistency when the model is fed new data.
☛ Use the model for prediction by feeding it new data, and use the coefficient of determination (R squared) as a model validity measure.
☛ Use data splitting to form a separate dataset for estimating model parameters, and another for validating predictions.
☛ Use jackknife resampling if the dataset contains a small number of instances, and measure validity with R squared and mean squared error (MSE).
13 :: Do you know what is the difference between rnorm and runif functions?
rnorm function-
Basically, it generates “n” normal random numbers. That is totally based on the mean and standard deviation arguments passed to the function.
Syntax of rnorm function –
rnorm(n, mean = , sd = )
runif function-
Basically, it generates “n” unform random numbers in the interval. That is of minimum and maximum values passed to the function.
Syntax of runif function –
runif(n, min = , max = )
Read MoreBasically, it generates “n” normal random numbers. That is totally based on the mean and standard deviation arguments passed to the function.
Syntax of rnorm function –
rnorm(n, mean = , sd = )
runif function-
Basically, it generates “n” unform random numbers in the interval. That is of minimum and maximum values passed to the function.
Syntax of runif function –
runif(n, min = , max = )
14 :: Explain me for loop control statement in R?
A loop is a sequence of instructions that is repeated until a certain condition is been reached. for, while and repeat, with the additional clauses break and next are used to construct loops.
For Example-
It is executed a known number of times for a block is been contained within curly braces.
x = c(1,2,3,4,5)
for(i in 1:5){
print(x[i])
}
[1] 1
[1] 2
[1] 3
[1] 4
[1] 5
Read MoreFor Example-
It is executed a known number of times for a block is been contained within curly braces.
x = c(1,2,3,4,5)
for(i in 1:5){
print(x[i])
}
[1] 1
[1] 2
[1] 3
[1] 4
[1] 5
15 :: Tell me you develop a big data model, but your end user has difficulty understanding how the model works and the insights it can reveal. How do you communicate with the user to get your points across?
Many big data analysts come from statistics, engineering, and computer science disciplines; they're brilliant analysts, but their people and communications skills lag. Businesses understand that to obtain results, you need both strong execution and strong communication. You can expect your HR, end business, and IT interviewers to focus on your communications skills, and to try to test them with a hypothetical situation.
Read More16 :: Tell me what cross-validation technique would you use on a time series dataset?
Instead of using k-fold cross-validation, you should be aware to the fact that a time series is not randomly distributed data - It is inherently ordered by chronological order.
In case of time series data, you should use techniques like forward chaining – Where you will be model on past data then look at forward-facing data.
fold 1: training[1], test[2]
fold 1: training[1 2], test[3]
fold 1: training[1 2 3], test[4]
fold 1: training[1 2 3 4], test[5]
Read MoreIn case of time series data, you should use techniques like forward chaining – Where you will be model on past data then look at forward-facing data.
fold 1: training[1], test[2]
fold 1: training[1 2], test[3]
fold 1: training[1 2 3], test[4]
fold 1: training[1 2 3 4], test[5]
17 :: Tell us why is resampling done?
Resampling is done in any of these cases:
☛ Estimating the accuracy of sample statistics by using subsets of accessible data or drawing randomly with replacement from a set of data points
☛ Substituting labels on data points when performing significance tests
☛ Validating models by using random subsets (bootstrapping, cross validation)
Read More☛ Estimating the accuracy of sample statistics by using subsets of accessible data or drawing randomly with replacement from a set of data points
☛ Substituting labels on data points when performing significance tests
☛ Validating models by using random subsets (bootstrapping, cross validation)
18 :: Tell me what methods do you use to identify outliers within a data set?
Data scientists must be able to go beyond classroom theoretical applications to real-world applications. Your candidate's answer to this question will show how they allocate their time to finding the best way to detect outliers. This information is important to know because it demonstrates the candidate's analytical skills. Look for answers that include:
☛ Raw data analysis
☛ Models
☛ Approaches
Read More☛ Raw data analysis
☛ Models
☛ Approaches
19 :: Can you describe strsplit() in R string manipulation?
Keywords
Character
Usage
strsplit(x, split, fixed = FALSE, perl = FALSE, useBytes = FALSE)
Arguments
a. x
It is a character vector, each element of which is to be split.
b. split
Basically, it is a character vector containing regular expression(s). That is used for splitting.
c. fixed
Since it is TRUE then it will match split exactly.
d. perl
Should Perl-compatible regexps be used?
e. useBytes
It is TRUE then the matching will do byte-by-byte rather than character-by-character, and inputs with marked encodings are not converted.
Read MoreCharacter
Usage
strsplit(x, split, fixed = FALSE, perl = FALSE, useBytes = FALSE)
Arguments
a. x
It is a character vector, each element of which is to be split.
b. split
Basically, it is a character vector containing regular expression(s). That is used for splitting.
c. fixed
Since it is TRUE then it will match split exactly.
d. perl
Should Perl-compatible regexps be used?
e. useBytes
It is TRUE then the matching will do byte-by-byte rather than character-by-character, and inputs with marked encodings are not converted.
20 :: Explain me nchar() in R string manipulation?
To find out if elements of a character vector are non-empty strings or not then nzchar is the fastest way.
Keywords
character
Usage
nchar(x, type = “chars”, allowNA = FALSE, keepNA = NA)
nzchar(x, keepNA = FALSE)
Arguments
a. x
Basically, a character vector or a vector will be restricted to a character vector. Giving a factor is an error.
b. type
character string: partial matching to one of c(“bytes”, “chars”, “width”).
c. allowNA
Should NA will return for invalid multibyte strings or “bytes”-encoded strings
d. keepNA
The default for nchar(), NA, means to use keepNA = TRUE unless type is “width”. Used to be hardcoded to FALSE in R versions ≤ 3.2.0.
Read MoreKeywords
character
Usage
nchar(x, type = “chars”, allowNA = FALSE, keepNA = NA)
nzchar(x, keepNA = FALSE)
Arguments
a. x
Basically, a character vector or a vector will be restricted to a character vector. Giving a factor is an error.
b. type
character string: partial matching to one of c(“bytes”, “chars”, “width”).
c. allowNA
Should NA will return for invalid multibyte strings or “bytes”-encoded strings
d. keepNA
The default for nchar(), NA, means to use keepNA = TRUE unless type is “width”. Used to be hardcoded to FALSE in R versions ≤ 3.2.0.
21 :: Tell me how do clean up and organize big data sets?
Data scientists frequently have to combine large amounts of information from various devices in several formats, such as data from a smartwatch or cellphone. Answers to this question will demonstrate how your candidate's methods for organizing large data. This information is important to know because data scientists need clean data to analyze information accurately to offer recommendations that solve business problems. Possible answers may include:
☛ Automation tools
☛ Value correction methods
☛ Comprehension of data sets
Read More☛ Automation tools
☛ Value correction methods
☛ Comprehension of data sets
22 :: Do you know more functions in brief in R?
read.spss Function – read.spss
What it does – Reads spss data file
For Example- spss(“myfile”)
read.xport Function – read.xport
What it does – Reads SAS export file
For Example- export(“myfile”)
read.dta Function – read.dta
What it does – Reads stata binary file
For Example – read.dta(“myfile”)
Read MoreWhat it does – Reads spss data file
For Example- spss(“myfile”)
read.xport Function – read.xport
What it does – Reads SAS export file
For Example- export(“myfile”)
read.dta Function – read.dta
What it does – Reads stata binary file
For Example – read.dta(“myfile”)
23 :: Tell me what is exploding gradients?
“Exploding gradients are a problem where large error gradients accumulate and result in very large updates to neural network model weights during training.” At an extreme, the values of weights can become so large as to overflow and result in NaN values.
This has the effect of your model being unstable and unable to learn from your training data. Now let’s understand what is the gradient.
Gradient:
Gradient is the direction and magnitude calculated during training of a neural network that is used to update the network weights in the right direction and by the right amount.
Read MoreThis has the effect of your model being unstable and unable to learn from your training data. Now let’s understand what is the gradient.
Gradient:
Gradient is the direction and magnitude calculated during training of a neural network that is used to update the network weights in the right direction and by the right amount.
24 :: Can you explain me what is logistic regression? Or State an example when you have used logistic regression recently?
Logistic Regression often referred as logit model is a technique to predict the binary outcome from a linear combination of predictor variables. For example, if you want to predict whether a particular political leader will win the election or not. In this case, the outcome of prediction is binary i.e. 0 or 1 (Win/Lose). The predictor variables here would be the amount of money spent for election campaigning of a particular candidate, the amount of time spent in campaigning, etc.
Read More25 :: What are the steps in making a decision tree?
☛ Take the entire data set as input.
☛ Look for a split that maximizes the separation of the classes. A split is any test that divides the data into two sets.
☛ Apply the split to the input data (divide step).
☛ Re-apply steps 1 to 2 to the divided data.
☛ Stop when you meet some stopping criteria.
☛ This step is called pruning. Clean up the tree if you went too far doing splits.
Read More☛ Look for a split that maximizes the separation of the classes. A split is any test that divides the data into two sets.
☛ Apply the split to the input data (divide step).
☛ Re-apply steps 1 to 2 to the divided data.
☛ Stop when you meet some stopping criteria.
☛ This step is called pruning. Clean up the tree if you went too far doing splits.