Data Scientist Interview Questions And Answers
Refine your Data Scientist interview skills with our 55 critical questions. Each question is crafted to challenge your understanding and proficiency in Data Scientist. Suitable for all skill levels, these questions are essential for effective preparation. Secure the free PDF to access all 55 questions and guarantee your preparation for your Data Scientist interview. This guide is crucial for enhancing your readiness and self-assurance.
55 Data Scientist Questions and Answers:
Data Scientist Job Interview Questions Table of Contents:
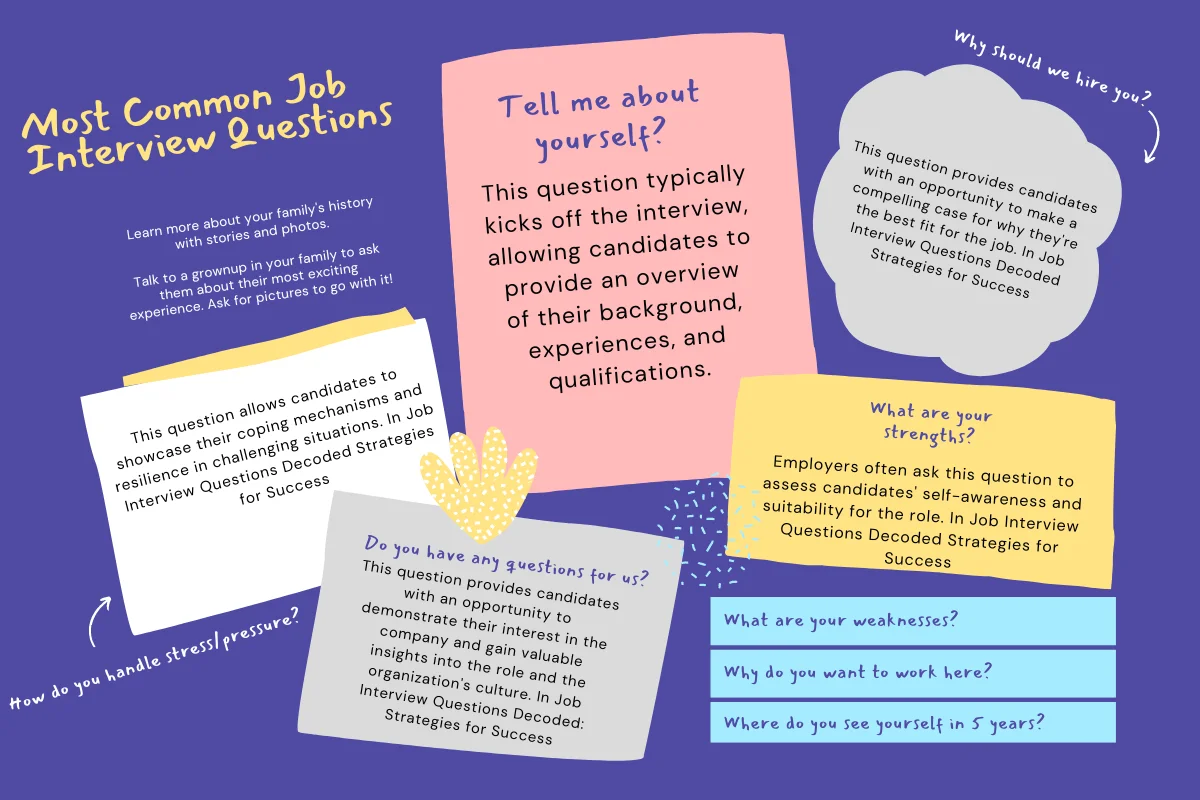
1 :: Tell me how do you handle missing or corrupted data in a dataset?
You could find missing/corrupted data in a dataset and either drop those rows or columns, or decide to replace them with another value.
In Pandas, there are two very useful methods:
isnull() and dropna() that will help you find columns of data with missing or corrupted data and drop those values. If you want to fill the invalid values with a placeholder value (for example, 0), you could use the fillna() method.
Read MoreIn Pandas, there are two very useful methods:
isnull() and dropna() that will help you find columns of data with missing or corrupted data and drop those values. If you want to fill the invalid values with a placeholder value (for example, 0), you could use the fillna() method.
2 :: Tell us why do we have max-pooling in classification CNNs?
Again as you would expect this is for a role in Computer Vision. Max-pooling in a CNN allows you to reduce computation since your feature maps are smaller after the pooling. You don’t lose too much semantic information since you’re taking the maximum activation. There’s also a theory that max-pooling contributes a bit to giving CNNs more translation in-variance.
Read More3 :: Tell us how do you identify a barrier to performance?
This question will determine how the candidate approaches solving real-world issues they will face in their role as a data scientist. It will also determine how they approach problem-solving from an analytical standpoint. This information is vital to understand because data scientists must have strong analytical and problem-solving skills. Look for answers that reveal:
Examples of problem-solving methods
Steps to take to identify the barriers to performance
Benchmarks for assessing performance
"My approach to determining performance bottlenecks is to conduct a performance test. I then evaluate the performance based on criteria set by the lead data scientist or company and discuss my findings with my team lead and group."
Read MoreExamples of problem-solving methods
Steps to take to identify the barriers to performance
Benchmarks for assessing performance
"My approach to determining performance bottlenecks is to conduct a performance test. I then evaluate the performance based on criteria set by the lead data scientist or company and discuss my findings with my team lead and group."
4 :: Tell us how do clean up and organize big data sets?
Data scientists frequently have to combine large amounts of information from various devices in several formats, such as data from a smartwatch or cellphone. Answers to this question will demonstrate how your candidate's methods for organizing large data. This information is important to know because data scientists need clean data to analyze information accurately to offer recommendations that solve business problems. Possible answers may include:
☛ Automation tools
☛ Value correction methods
☛ Comprehension of data sets
Read More☛ Automation tools
☛ Value correction methods
☛ Comprehension of data sets
5 :: Explain me do gradient descent methods at all times converge to a similar point?
No, they do not because in some cases they reach a local minima or a local optima point. You would not reach the global optima point. This is governed by the data and the starting conditions.
Read More6 :: Tell me why is resampling done?
Resampling is done in any of these cases:
☛ Estimating the accuracy of sample statistics by using subsets of accessible data or drawing randomly with replacement from a set of data points
☛ Substituting labels on data points when performing significance tests
☛ Validating models by using random subsets (bootstrapping, cross validation)
Read More☛ Estimating the accuracy of sample statistics by using subsets of accessible data or drawing randomly with replacement from a set of data points
☛ Substituting labels on data points when performing significance tests
☛ Validating models by using random subsets (bootstrapping, cross validation)
7 :: Tell me how is kNN different from kmeans clustering?
Don’t get mislead by ‘k’ in their names. You should know that the fundamental difference between both these algorithms is, kmeans is unsupervised in nature and kNN is supervised in nature. kmeans is a clustering algorithm. kNN is a classification (or regression) algorithm.
kmeans algorithm partitions a data set into clusters such that a cluster formed is homogeneous and the points in each cluster are close to each other. The algorithm tries to maintain enough separability between these clusters. Due to unsupervised nature, the clusters have no labels.
Read Morekmeans algorithm partitions a data set into clusters such that a cluster formed is homogeneous and the points in each cluster are close to each other. The algorithm tries to maintain enough separability between these clusters. Due to unsupervised nature, the clusters have no labels.
8 :: Can you differentiate between univariate, bivariate and multivariate analysis?
These are descriptive statistical analysis techniques which can be differentiated based on the number of variables involved at a given point of time. For example, the pie charts of sales based on territory involve only one variable and can be referred to as univariate analysis.
If the analysis attempts to understand the difference between 2 variables at time as in a scatterplot, then it is referred to as bivariate analysis. For example, analysing the volume of sale and a spending can be considered as an example of bivariate analysis.
Analysis that deals with the study of more than two variables to understand the effect of variables on the responses is referred to as multivariate analysis.
Read MoreIf the analysis attempts to understand the difference between 2 variables at time as in a scatterplot, then it is referred to as bivariate analysis. For example, analysing the volume of sale and a spending can be considered as an example of bivariate analysis.
Analysis that deals with the study of more than two variables to understand the effect of variables on the responses is referred to as multivariate analysis.
9 :: Tell me how can outlier values be treated?
Outlier values can be identified by using univariate or any other graphical analysis method. If the number of outlier values is few then they can be assessed individually but for large number of outliers the values can be substituted with either the 99th or the 1st percentile values. All extreme values are not outlier values.The most common ways to treat outlier values –
☛ 1) To change the value and bring in within a range
☛ 2) To just remove the value.
Read More☛ 1) To change the value and bring in within a range
☛ 2) To just remove the value.
10 :: Explain me what is data normalization and why do we need it?
I felt this one would be important to highlight. Data normalization is very important preprocessing step, used to rescale values to fit in a specific range to assure better convergence during backpropagation. In general, it boils down to subtracting the mean of each data point and dividing by its standard deviation. If we don’t do this then some of the features (those with high magnitude) will be weighted more in the cost function (if a higher-magnitude feature changes by 1%, then that change is pretty big, but for smaller features it’s quite insignificant). The data normalization makes all features weighted equally.
Read More11 :: Tell us why do we use convolutions for images rather than just FC layers?
This one was pretty interesting since it’s not something companies usually ask. As you would expect, I got this question from a company focused on Computer Vision. This answer has 2 parts to it. Firstly, convolutions preserve, encode, and actually use the spatial information from the image. If we used only FC layers we would have no relative spatial information. Secondly, Convolutional Neural Networks (CNNs) have a partially built-in translation in-variance, since each convolution kernel acts as it’s own filter/feature detector.
Read More12 :: Tell us how has your prior experience prepared you for a role in data science?
This question helps determine the candidate's experience from a holistic perspective and reveals experience in demonstrating interpersonal, communication and technical skills. It is important to understand this because data scientists must be able to communicate their findings, work in a team environment and have the skills to perform the task. Here are some possible answers to look for:
☛ Project management skills
☛ Examples of working in a team environment
☛ Ability to identify errors
Read More☛ Project management skills
☛ Examples of working in a team environment
☛ Ability to identify errors
13 :: Do you know what is logistic regression?
Logistic Regression is also known as the logit model. It is a technique to forecast the binary outcome from a linear combination of predictor variables.
Read More14 :: Tell us how regularly must an algorithm be updated?
You will want to update an algorithm when:
☛ You want the model to evolve as data streams through infrastructure
☛ The underlying data source is changing
☛ There is a case of non-stationarity
Read More☛ You want the model to evolve as data streams through infrastructure
☛ The underlying data source is changing
☛ There is a case of non-stationarity
15 :: Do you know why is naive Bayes so ‘naive’ ?
naive Bayes is so ‘naive’ because it assumes that all of the features in a data set are equally important and independent. As we know, these assumption are rarely true in real world scenario.
Read More16 :: Explain me why data cleaning plays a vital role in analysis?
Cleaning data from multiple sources to transform it into a format that data analysts or data scientists can work with is a cumbersome process because - as the number of data sources increases, the time take to clean the data increases exponentially due to the number of sources and the volume of data generated in these sources. It might take up to 80% of the time for just cleaning data making it a critical part of analysis task.
Read More17 :: Do you know what is the goal of A/B Testing?
It is a statistical hypothesis testing for randomized experiment with two variables A and B. The goal of A/B Testing is to identify any changes to the web page to maximize or increase the outcome of an interest. An example for this could be identifying the click through rate for a banner ad.
Read More18 :: What is dimensionality reduction, where it’s used, and it’s benefits?
Dimensionality reduction is the process of reducing the number of feature variables under consideration by obtaining a set of principal variables which are basically the important features. Importance of a feature depends on how much the feature variable contributes to the information representation of the data and depends on which technique you decide to use. Deciding which technique to use comes down to trial-and-error and preference. It’s common to start with a linear technique and move to non-linear techniques when results suggest inadequate fit.
Benefits of dimensionality reduction for a data set may be:
(1) Reduce the storage space needed
(2) Speed up computation (for example in machine learning algorithms), less dimensions mean less computing, also less dimensions can allow usage of algorithms unfit for a large number of dimensions
(3) Remove redundant features, for example no point in storing a terrain’s size in both sq meters and sq miles (maybe data gathering was flawed)
(4) Reducing a data’s dimension to 2D or 3D may allow us to plot and visualize it, maybe observe patterns, give us insights
(5) Too many features or too complex a model can lead to overfitting.
Read MoreBenefits of dimensionality reduction for a data set may be:
(1) Reduce the storage space needed
(2) Speed up computation (for example in machine learning algorithms), less dimensions mean less computing, also less dimensions can allow usage of algorithms unfit for a large number of dimensions
(3) Remove redundant features, for example no point in storing a terrain’s size in both sq meters and sq miles (maybe data gathering was flawed)
(4) Reducing a data’s dimension to 2D or 3D may allow us to plot and visualize it, maybe observe patterns, give us insights
(5) Too many features or too complex a model can lead to overfitting.
19 :: Explain me what tools or devices help you succeed in your role as a data scientist?
This question's purpose is to learn the programming languages and applications the candidate knows and has experience using. The answer will show the candidate's need for additional training of basic programming languages and platforms or any transferable skills. This is vital to understand as it can cost more time and money to train if the candidate is not knowledgeable in all of the languages and applications required for the position. Answers to look for include:
☛ Experience in SAS and R programming
☛ Understanding of Python, PHP or Java programming languages
☛ Experience using data visualization tools
"I believe I can excel in this position with my R, Python, and SQL programming skill set. I enjoy working on the FUSE and Tableau platforms to mine data and draw inferences."
Read More☛ Experience in SAS and R programming
☛ Understanding of Python, PHP or Java programming languages
☛ Experience using data visualization tools
"I believe I can excel in this position with my R, Python, and SQL programming skill set. I enjoy working on the FUSE and Tableau platforms to mine data and draw inferences."
20 :: What is star schema?
It is a traditional database schema with a central table. Satellite tables map IDs to physical names or descriptions and can be connected to the central fact table using the ID fields; these tables are known as lookup tables and are principally useful in real-time applications, as they save a lot of memory. Sometimes star schemas involve several layers of summarization to recover information faster.
Read More21 :: Tell me is rotation necessary in PCA? If yes, Why? What will happen if you don’t rotate the components?
Yes, rotation (orthogonal) is necessary because it maximizes the difference between variance captured by the component. This makes the components easier to interpret. Not to forget, that’s the motive of doing PCA where, we aim to select fewer components (than features) which can explain the maximum variance in the data set. By doing rotation, the relative location of the components doesn’t change, it only changes the actual coordinates of the points.
If we don’t rotate the components, the effect of PCA will diminish and we’ll have to select more number of components to explain variance in the data set.
Read MoreIf we don’t rotate the components, the effect of PCA will diminish and we’ll have to select more number of components to explain variance in the data set.
22 :: Explain me what is logistic regression? Or State an example when you have used logistic regression recently?
Logistic Regression often referred as logit model is a technique to predict the binary outcome from a linear combination of predictor variables. For example, if you want to predict whether a particular political leader will win the election or not. In this case, the outcome of prediction is binary i.e. 0 or 1 (Win/Lose). The predictor variables here would be the amount of money spent for election campaigning of a particular candidate, the amount of time spent in campaigning, etc.
Read More23 :: Explain me why do you want to work at this company as a data scientist?
The purpose of this question is to determine the motivation behind the candidate's choice of applying and interviewing for the position. Their answer should reveal their inspiration for working for the company and their drive for being a data scientist. It should show the candidate is pursuing the position because they are passionate about data and believe in the company, two elements that can determine the candidate's performance. Answers to look for include:
☛ Interest in data mining
☛ Respect for the company's innovative practices
☛ Desire to apply analytical skills to solve real-world issues with data
Read More☛ Interest in data mining
☛ Respect for the company's innovative practices
☛ Desire to apply analytical skills to solve real-world issues with data
24 :: Tell us how would you go about doing an Exploratory Data Analysis (EDA)?
The goal of an EDA is to gather some insights from the data before applying your predictive model i.e gain some information. Basically, you want to do your EDA in a coarse to fine manner.
We start by gaining some high-level global insights. Check out some imbalanced classes. Look at mean and variance of each class. Check out the first few rows to see what it’s all about. Run a pandas df.info() to see which features are continuous, categorical, their type (int, float, string).
Next, drop unnecessary columns that won’t be useful in analysis and prediction. These can simply be columns that look useless, one’s where many rows have the same value (i.e it doesn’t give us much information), or it’s missing a lot of values. We can also fill in missing values with the most common value in that column, or the median. Now we can start making some basic visualizations. Start with high-level stuff. Do some bar plots for features that are categorical and have a small number of groups. Bar plots of the final classes. Look at the most “general features”.
Create some visualizations about these individual features to try and gain some basic insights. Now we can start to get more specific.
Create visualizations between features, two or three at a time. How are features related to each other? You can also do a PCA to see which features contain the most information. Group some features together as well to see their relationships. For example, what happens to the classes when A = 0 and B = 0? How about A = 1 and B = 0? Compare different features. For example, if feature A can be either “Female” or “Male” then we can plot feature A against which cabin they stayed in to see if Males and Females stay in different cabins.
Beyond bar, scatter, and other basic plots, we can do a PDF/CDF, overlayed plots, etc. Look at some statistics like distribution, p-value, etc. Finally it’s time to build the ML model. Start with easier stuff like Naive Bayes and Linear Regression. If you see that those suck or the data is highly non-linear, go with polynomial regression, decision trees, or SVMs. The features can be selected based on their importance from the EDA. If you have lots of data you can use a Neural Network. Check ROC curve. Precision, Recall
Read MoreWe start by gaining some high-level global insights. Check out some imbalanced classes. Look at mean and variance of each class. Check out the first few rows to see what it’s all about. Run a pandas df.info() to see which features are continuous, categorical, their type (int, float, string).
Next, drop unnecessary columns that won’t be useful in analysis and prediction. These can simply be columns that look useless, one’s where many rows have the same value (i.e it doesn’t give us much information), or it’s missing a lot of values. We can also fill in missing values with the most common value in that column, or the median. Now we can start making some basic visualizations. Start with high-level stuff. Do some bar plots for features that are categorical and have a small number of groups. Bar plots of the final classes. Look at the most “general features”.
Create some visualizations about these individual features to try and gain some basic insights. Now we can start to get more specific.
Create visualizations between features, two or three at a time. How are features related to each other? You can also do a PCA to see which features contain the most information. Group some features together as well to see their relationships. For example, what happens to the classes when A = 0 and B = 0? How about A = 1 and B = 0? Compare different features. For example, if feature A can be either “Female” or “Male” then we can plot feature A against which cabin they stayed in to see if Males and Females stay in different cabins.
Beyond bar, scatter, and other basic plots, we can do a PDF/CDF, overlayed plots, etc. Look at some statistics like distribution, p-value, etc. Finally it’s time to build the ML model. Start with easier stuff like Naive Bayes and Linear Regression. If you see that those suck or the data is highly non-linear, go with polynomial regression, decision trees, or SVMs. The features can be selected based on their importance from the EDA. If you have lots of data you can use a Neural Network. Check ROC curve. Precision, Recall
25 :: Tell me why do segmentation CNNs typically have an encoder-decoder style / structure?
The encoder CNN can basically be thought of as a feature extraction network, while the decoder uses that information to predict the image segments by “decoding” the features and upscaling to the original image size.
Read More