Data Scientist Question:
Explain me when is Ridge regression favorable over Lasso regression?
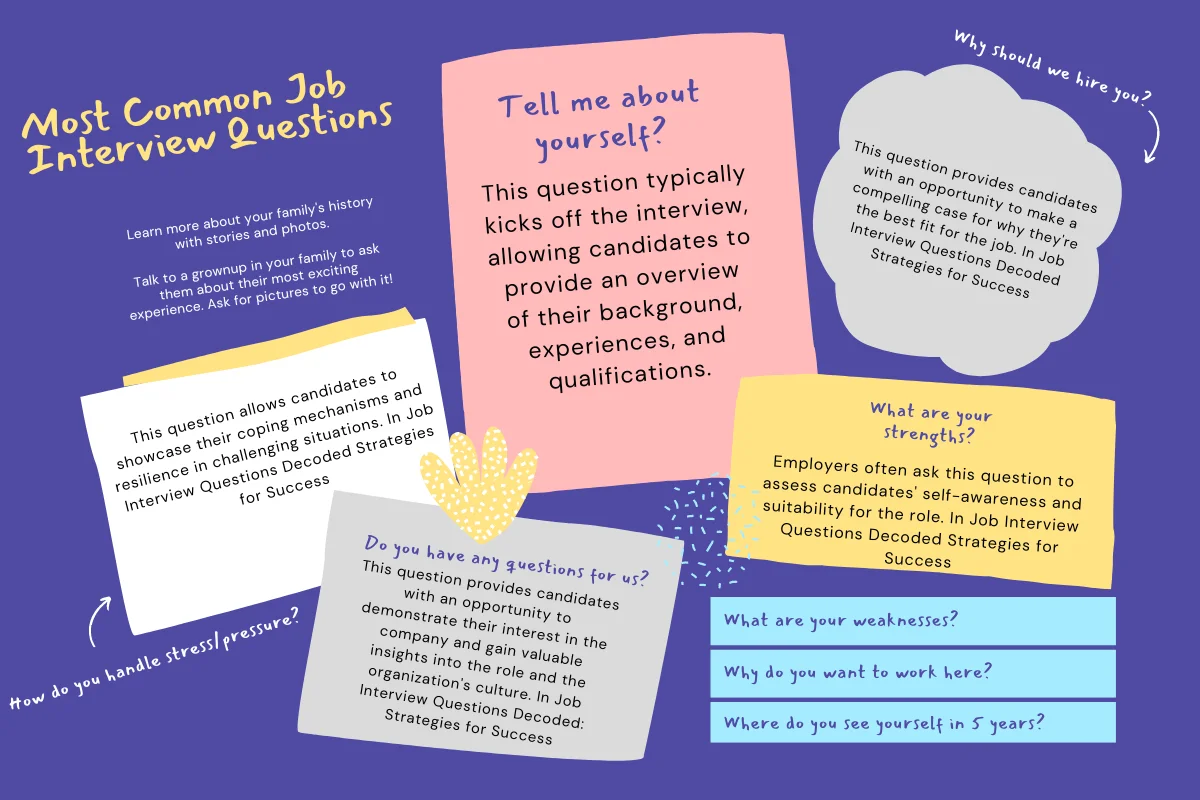
Answer:
You can quote ISLR’s authors Hastie, Tibshirani who asserted that, in presence of few variables with medium / large sized effect, use lasso regression. In presence of many variables with small / medium sized effect, use ridge regression.
Conceptually, we can say, lasso regression (L1) does both variable selection and parameter shrinkage, whereas Ridge regression only does parameter shrinkage and end up including all the coefficients in the model. In presence of correlated variables, ridge regression might be the preferred choice. Also, ridge regression works best in situations where the least square estimates have higher variance. Therefore, it depends on our model objective.
Conceptually, we can say, lasso regression (L1) does both variable selection and parameter shrinkage, whereas Ridge regression only does parameter shrinkage and end up including all the coefficients in the model. In presence of correlated variables, ridge regression might be the preferred choice. Also, ridge regression works best in situations where the least square estimates have higher variance. Therefore, it depends on our model objective.
Previous Question | Next Question |
Tell me how do you work towards a random forest? | Tell me what is power analysis? |